Table Of Contents
What Is A Covariate?
Covariate, in statistics, is a variable that is related to both the independent variable(s) and the dependent variable in a research study or statistical analysis. It's used to account for the potential influence of that variable on the dependent variable.
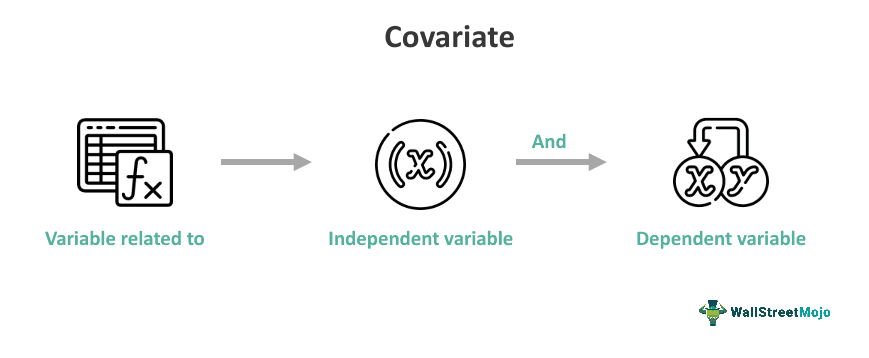
Covariates are used to control for the potential influence of other variables that might confound or distort the relationship between the independent variable(s) and the dependent variable. In research and statistical analysis, covariates are used to reduce confounding of variations that are not of primary interest in the study.
Key Takeaways
- A covariate is a variable used in statistical analysis that is related to both the independent and the dependent variables.
- They help researchers better understand and interpret the relationship between both variables by factoring in the influence of other relevant variables.
- These are variables related to both dependent and independent variables, whereas the moderator influences the strength of the relationship between both variables.
- A confounding variable is similar to a covariate, but these create a spurious relation between them.
- Factor more often refers to the variable that is used in a research study. In contrast, a confounder creates a misleading interpretation relationship between the two variables.
Covariate In Statistics Explained
Covariate in statistics is a fundamental metric used to assess the relationship between two random variables. Besides, it quantifies the degree to which these variables change together. Essentially, covariance measures the joint variability of these variables. However, it's important to note that covariance, by itself, does not indicate the exact nature of the dependency between the variables.
Furthermore, the covariate in regression represents the change in the dependent variable for a one-unit change in the corresponding independent variable. Hence, binary covariates are commonly used in regression models. Therefore, these play a specific role in understanding the relationship between the independent variable(s) and the dependent variable.
Interpreting covariance values is critical to understanding the relationship between the variables. A positive covariance suggests that the two variables tend to move in the same direction. Meaning when one increases, the other tends to increase as well, and vice versa. On the other hand, a negative covariance indicates that the variables tend to move in opposite directions, with one increasing when the other decreases. Hence, the goal of covariate analysis is to understand how these additional variables may impact the relationship between the variables of primary interest.
The formula is -
Cov(X, Y) = Σ / N-1
Where:
- Cov(X, Y) represents the covariance between variables X and Y.
- Σ denotes the summation symbol, indicating that you should sum the following expression for each data point.
- Xáµ¢ and Yáµ¢ are individual data points from the datasets X and Y
- μₓ is the mean of dataset X.
- μᵧ is the mean of dataset Y.
- N is the total number of data points in the datasets.
This formula calculates the average of the product of the differences between individual data points and their respective means for both variables.
Examples
Let us look at the covariate examples to understand the concept better -
Example #1
In this scenario, we have Rosy, an employee at a software company. Therefore, the goal is to analyze the factors influencing Rosy's job performance measured by her monthly project completion rate. The variables of interest are her daily work hours and her stress level.
- Dependent Variable: Monthly Project Completion Rate (%)
- Independent Variable of Interest: Daily Work Hours
Now, introducing the covariate:
Covariate: Stress Level (measured on a scale from 1 to 10, with one being low stress and 10 being high stress)
Rosy's stress level might impact her job performance. The question is whether, when controlling for stress level, her daily work hours continue to affect her project completion rate significantly.
The analysis provides several insights:
- Effect of Daily Work Hours
- Effect of Stress Level
- Interaction
Example #2
On May 30, 2023, the US Food and Drug Administration (FDA) published a final advice on how sponsors can account for covariates when analyzing controlled clinical trials for pharmaceuticals and biological products.
According to the FDA, "the primary emphasis of the recommendations is on the use of predictive foundation covariates. Thus, to enhance the accuracy of statistics for estimating and testing treatment effects." It offers broad guidelines for covariate changes in both linear and nonlinear models.
Therefore, when estimating the treatment effects of a new medicine in many controlled studies, the initial analysis may need to account for baseline factors. Moreover, the FDA notes in the guidance that including prognostic baseline factors in the design and analysis of clinical trial data can lead to a more effective use of data to illustrate and measure the effects of treatment.
These recommendations don't cover using covariates to account for confounding factors in non-randomized trials. But instead, they use covariates to account for missing outcome data or using Bayesian approaches to account for covariate adjustment.
Covariate vs Confounder vs Factor
The differences between covariate, confounder, and factor are as follows:
Aspects | Covariate | Confounder | Factor |
---|---|---|---|
Meaning | A covariate is a variable that is related to both the independent variable(s) and the dependent variable in a research study or statistical analysis. | The confounder is a specific type of covariate that is associated with both the exposure (independent variable) and the outcome (dependent variable) and can distort or falsely indicate a relationship between them. | The term "factor" is more general and can refer to any variable or element that is considered in a research study. |
Role | These are used to control for its effects to improve the precision of the estimates of primary variables. | Needs to be controlled for because it may distort the relationship between the independent variable of interest and the dependent variable. | Here, the variable is intentionally manipulated or categorized to observe its effect on the dependent variable. |
Purpose | Control for potential sources of variability and increase the accuracy of estimates. | Identify and control for potential sources of bias in the relationship between the independent and dependent variables. | Assess the impact of different levels or categories of the variable on the dependent variable. |
Covariate vs Moderator vs Confounding Variable
The differences between covariate, moderator, and confounding variable are as follows -
Aspects | Covariate | Moderator | Confounding variable |
---|---|---|---|
Meaning | A confounding variable is a variable that is related to both the independent variable and the dependent variable, and it can create a false or misleading association between them. | A moderator is a variable that influences the strength or direction of the relationship between an independent variable and a dependent variable. | Confounding variable is a variable that is related to both the independent variable and the dependent variable, and it can create a false or misleading association between them. |
Purpose | Enhances the accuracy of estimates by controlling for potential sources of variability. | Investigate under what conditions or for whom the relationship between the independent and dependent variables changes. | Identify and eliminate the impact of a third variable that could mistakenly be attributed to the relationship between the independent and dependent variables. |
Role | A covariate is a variable that exhibits associations or connections with both the independent variable(s) and the dependent variable in a research study or statistical analysis. | Examines the conditions under which an independent variable is related to a dependent variable.. | Introduces bias by affecting the observed relationship between the independent and dependent variables. |