Table Of Contents
Copula Meaning
Copula is a probabilistic model that analyzes the relationship between multiple variables by separating their joint and marginal probabilities. It plays a significant role in finance, particularly in portfolio credit asset allocation, options pricing, and financial risk management.
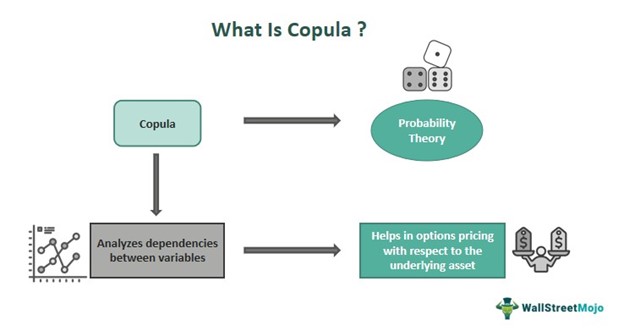
This probability helps quantify dependence within complex multivariate systems, offering insights into their interconnections and dependencies. It enables modeling and understanding default dependence structures among credit assets, aiding in optimal portfolio composition. They also help in assessing systemic risks in the financial market, leading to the implementation of improved risk estimation and prevention measures.
Key Takeaways
- Copula is a statistical tool used to model the dependence structure between multiple variables without assuming individual distributions or relationship forms.
- It is applied in finance, insurance, and risk management to model dependencies between variables without assuming specific distributions.
- It helps understand complex relationships, assess risk, and optimize portfolio allocation.
- Unlike correlation, this model provides a more flexible and comprehensive approach to modeling interconnections, making it valuable in various fields.
Copula In Finance Explained
Copula theory is a widely used method for analyzing dependencies between variables, particularly in risk and survival applications. It helps investigate tail dependencies and is crucial in economic and financial modeling for predicting events like financial contagion. In financial modeling, the choice of this model is pivotal, as it determines how extreme events are represented in the data set tails.
The modeling process begins with identifying and determining the distributions of the variables. These are then transformed into marginal distributions, typically uniform. Finally, a suitable model is selected to represent the dependencies between the variables, allowing for the analysis of extreme events within the dataset.
The history of this modeling technique in finance includes criticism of the Gaussian copula for its role in the 2007-2008 financial crisis. However, the blame lies with the modelers who failed to specify the model properly. Parametric modeling involves a systematic process, from identifying distributions to selecting appropriate models, with numerous examples available in its modeling literature.
Examples
Let us look at these examples to understand the copula model better.
Example #1
Consider Richard, an investor managing a diversified portfolio who employs copula modeling to examine the relationship between stock returns and bond yields. He examines historical data to differentiate how stock returns and bond yields co-move. By fitting a copula to the dataset, he ascertains the joint distribution of these variables, capturing any nonlinear relationships or tail dependencies.
During periods of economic uncertainty, Richard notices that stock returns tend to be negatively correlated with bond yields, reflecting a flight to safety among investors. Conversely, during economic expansions, he observes a positive correlation between stock returns and bond yields as investors pursue higher returns.
Gaining insights, Richard adjusts his portfolio composition. He hedges against potential risks and seizes opportunities based on the observed dependencies between stocks and bonds.
Example #2
A study conducted between 1987 and 2017 in Ethiopia's Guder basin used copula modeling to model flood peak and volume characteristics. The research aimed to improve understanding of flood event features essential for hydraulic and hydrological system design. By employing four Archimedean copulas and executing graphical and quantifiable tests, the Gumbel-Hougaard copula emerged as the best fit for the data. This finding emphasized the usefulness of copula modeling in examining joint flood features.
Additionally, the study calculated the bivariate probability and return periods for the volume and peak of the flood. One conducts these calculations separately as well as in the AND and OR states. It helps in understanding the likelihood of simultaneously experiencing a large flood peak and an enormous volume of water. This data is crucial for organizing flood management strategies and developing infrastructure that decreases their effects. Copula methods have been an excellent tool for handling individual flood-related factors and predicting flood risk.
Applications In Finance
The most common application of the Copula probability model in finance is in the field of options pricing. The prices of the options are based on the fluctuations in the prices of the underlying securities. This pricing of the options marks the dependencies between variables, thereby indicating the relevance of this model here.
In portfolio analysis and risk management within financial markets, the copula function serves as a valuable tool. It helps in determining non-parametric measures of dependence between random variables. This makes it particularly suitable for sectors like banking and insurance. As global financial markets evolve and integrate, the interconnectedness between different markets increases. This amplifies the potential impact of market risk transmission across borders.
With economic globalization and expanding financial market openness, the interlinkages between markets worldwide strengthen. Variations in market openness between countries can worsen financial market turmoil. Fluctuations in one market affect closely related markets and potentially trigger major financial crises.
Given the distinct characteristics of financial asset income distribution, characterized by sharp peaks and thick tails, establishing dynamic nonlinear models becomes essential. These models depict the evolving correlation structure accurately. Copula functions emerge as a widely utilized nonlinear correlation research tool in financial risk analysis. They offer flexibility in capturing the nonlinear patterns and distinct distribution features of financial assets.
Copula vs Correlation
Copula
- This model focuses on modeling the dependence structure between variables without assuming their distributions or the form of their relationship. It captures any type of dependence, not just linear.
- This modeling does not produce a single summary statistic like correlation. Instead, it models the joint distribution of variables, allowing for a deeper understanding of their dependence structure and the ability to simulate joint outcomes.
Correlation
- Correlation measures the strength and direction of linear association between two variables. It indicates the extent to which one variable changes to the changes in the other variable, assuming a linear relationship.
- Correlation is limited to measuring linear relationships, which means it may not capture complex dependencies that exist in real-world scenarios.