Table Of Contents
What Is Chi Square Test?Â
The chi-square or chi-squared test is a statistical test used to find the relationship between the observed values and the expected values of raw variables. These values are random, independent, and mutually exclusive of the categorical dataset within a given distribution. It helps the researchers to check the degree to which observed data fits within a population of independent variables.
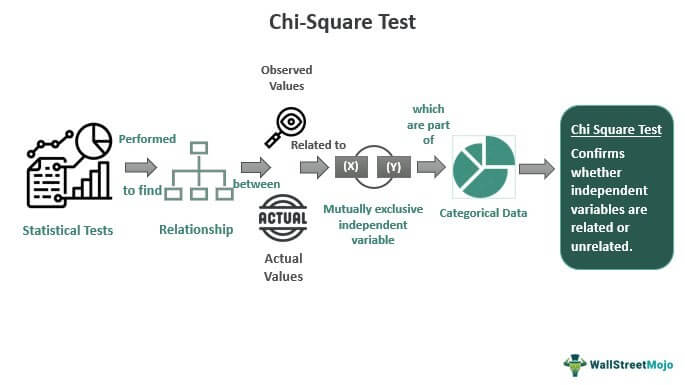
The test does not work with continuous non-categorical data. It is a good tool to measure the null hypothesis related to the independence of variables. Researchers can also test the probability of independence using the test. A P-value can be determined using this test if the researchers know the the degrees of freedom.
Chi Square Test ExplainedÂ
The Chi square test determines and compares the discrepancies in the relationship between observed and expected frequencies of independent mutually exclusive variables of categorical data in a population. It is symbolically represented as X2. It's also known as Pearson's Chi-square test, introduced in 1900 by mathematician Karl Pearson.
Whenever the variables remain independent and are nominal, the statistician undertakes the Chi-Square test to evaluate how well the measured data for a particular distribution matches the theoretical data. In short, it compares two statistical data sets. In addition, this test determines the resemblance between the values obtained and the expected values.
Further, it determines if the variables are related or independently distributed. So, it is sometimes referred to as a test of independence. It is the best experiment to prove or disprove a hypothesis. Researchers use another chi-square test type known as the 'goodness of fit' for a single measurement variable. It decides if a single variable is probable to come from a given distribution or not.
Verifying any of the two or more mutually exclusive propositions is necessary. The observations would tend to match the independent variable distributions exactly, according to the null hypothesis. So, it shows that the information that was seen was not skewed. An alternative theory proposes that observed data deviates from exogenous variable distributions, skewing the data or dependent variables.
The chi square test SPSS is the most widely used software for calculating the chi square test in r and chi square test p-value for a categorical data set distribution containing mutually exclusive and independent variables.
Chi-Squared DistributionÂ
Statisticians frequently utilize the Chi-Square distribution to assess how closely an actual distribution matches a theoretical one. Moreover, statisticians use degrees of freedom to quantify this metric. For example, researchers may use the Chi-Square distributions to determine the differences between the observed standard deviation of a population and a sample standard deviation with the setting of confidence intervals.
The Chi-Square distribution, in brief, simulates the total of the squares' spread of multiple independent normalized random variables. Therefore, as a result, by square rooting a conventional normal random variable X, one may obtain the Chi-Square distributions in their most basic form as:
Q1​= X2
Suppose one plot the function as mentioned earlier. In that case, one obtains a curve that rapidly decreases as q rises to practically zero. The distribution's values q are square values of random selections from a normally distributed populace. Most drawings would be near 0 since the average of the common normal distribution is equal to zero.
Chi-Square Test Properties
The properties of the chi-square test are the following:
- The variance equals two times the number of degrees of freedom
- The degree of freedom number is equal to the mean distribution.
- As the degree of freedom increases, the chi-square distribution curve approaches the normal distribution.
Formula
Let us understand the chi square test formula in the following section:
By employing the observed and predicted frequency, the Chi-Square function illustrates or tests the connections between the variables of two categories.
Let us represent chi square with χc2, then χ2= ∑(Oi – Ei)2/Ei.
Where:
- c = Degrees of freedom
- Oi = Observed or measured value.
- Ei = expected value.
The chi square test calculator or formula calculates the p-value for measuring the interdependence between two variables. First, users consider the possibility that a certain condition or assertion is valid, which users may then test. For instance:
- The gathered data shows very good agreement with the predicted statistics, according to a very tiny Chi-Square statistical test.
- According to a very tiny Chi-Square statistical test, the collected data shows very good agreement with the predicted statistics. However, the Chi-Square test value is relatively large, indicating that the data may not correlate well enough. As a result, the null hypothesis is disproved if the chi-square value is high.
Statisticians use this statistical test to find the P-value. A P-value is said to be the abbreviation of a probability value. It specifies the likelihood of obtaining an otherwise more excessive outcome than the other observed data or about the same as them. Or if it indicates the likelihood that the specified event will occur. Considering observed frequency versus predicted frequency, the lower the P, the greater the support is in line with the alternative hypothesis, as shown below.
- If the P-value is 0.05, then the null hypothesis is rejected.
- In conditions where the P-value is greater than 0.05, the null hypothesis is said to be accepted or impossible to be rejected.
- If the P-value exceeds 0.05, one should consider the null hypothesis more.
Test Of Independence
Statisticians utilize the statistical hypothesis test, known as the Chi-square test of independence, to examine if two independent or categorical variables have a higher probability of being connected or not. If one has counts of values across two categorical variables, one may apply this test. Additionally, when users believe there is no connection between the two variables, they can determine whether or not their proposal is feasible by using the test.
As an interpretive statistical test, this chi-square test of independence enables researchers to deduce information related to a population from a given sample. It allows users to determine whether or not the two given variables have a relationship within the populace. Moreover, the chi-square test of independence analyses a null plus an alternative hypothesis, as do all other hypothesis tests. The conflicting hypotheses address the issue of whether or not variable "or" variable two are connected as per the given template:
- Null hypothesis (H0) interpretation: Variable A and variable B do not correlate in the population since the ratios of variable A are the same as various values of variable B.
- Alternative hypothesis (Ha) interpretation: Variable A and variable B correlate with each other in the population since the proportions of variable A fluctuate depending on the value of variable B.
Also, the actual and anticipated frequencies are contrasted in a chi-square statistical test of independence. The ratios of one variable remain constant across the full values of the supplementary variable due to the predicted frequencies. Furthermore, one could use the contingency table to determine the anticipated frequencies. Suppose for a row r plus column c, the anticipated or expected frequency is:
Total of row r * a total of column c / N
Examples
The following examples help to understand the concept of the chi-square test:
Example #1
- An example of a chi square goodness of fit test is a decision if a sack of sports equipment has the same number of cricket balls of each color or not. Here, H0 = Proportion of the color of cricket balls is the same. But, on the other hand, ha = Proportion of color is not the same.
- The Chi-square test of independence example is to decide if college students' marks are related to the color of the clothes they wear. Here, H0 = Proportion of the marks depends on the clothes color. On the other hand, ha = Proportion of the marks is independent of the clothes color.
Example #2
Let us take the help of the chi square test table to understand the next example related to air-borne diseases in four countries and conduct a chi-square test to find the p-value.
Observed Frequency -
COUNTRIES | AIR BORNE DISEASE 1 | DISEASE 2 | DISEASE 3 | DISEASE 4 |
---|---|---|---|---|
ASTHMA | SINUS | COUGH | Total | |
USA | 21 | 32 | 40 | 93 |
UK | 10 | 12 | 31 | 53 |
CHINA | 15 | 10 | 12 | 37 |
Total | 46 | 54 | 83 | 183 |
N | 50 |
Expected Frequencies (Variables Perfectly Independent) -
COUNTRIES | AIR BORNE DISEASE 1 | DISEASE 2 | DISEASE 3 | DISEASE 4 |
---|---|---|---|---|
ASTHMA | SINUS | COUGH | Total | |
USA | 19 | 21 | 30 | 70 |
UK | 12 | 10 | 26 | 48 |
CHINA | 11 | 8 | 20 | 39 |
Total | 42 | 39 | 76 | 157 |
Chi-Square Points= (Observed (Oi)-Expected (Ei)) ^2/ Expected (Ei) -
COUNTRIES | AIR BORNE DISEASE 1 | DISEASE 2 | DISEASE 3 | DISEASE 4 |
---|---|---|---|---|
OBSERVED | EXPECTED | (Oi – Ei)2 | (Oi – Ei)2/Ei | |
USA | 93 | 70 | 529 | 7.557142857 |
UK | 53 | 48 | 25 | 0.520833333 |
CHINA | 37 | 39 | 4 | 0.102564103 |
Total | ||||
CHI-SQUARE | 8.180540293 | |||
Critical Value of Chi-square = | 7.814727903 |
Chi-Test (P)Value = | 0.002817847 |
The p-value <0.05, so one can consider the null hypothesis as rejected.
Chi Square Degrees Of FreedomÂ
The degrees of freedom correspond to the quantity of independent & random elements that constitute the Chi-Square distribution (df). Moreover, degrees of freedom are not explicitly defined. However, one may conceive of it as the number of variables that could fluctuate. One can get more degrees of freedom as one keeps adding variables since they increase variability. Larger sample size results in reliable data.
Hence, one could see how the Chi-Square distribution's df varies depending on the degrees of freedom throughout the accompanying plot. Most of the data would then cluster near elevated q (or) values, as one is squaring the results of k, which researchers draw from a random, normally distributed population.
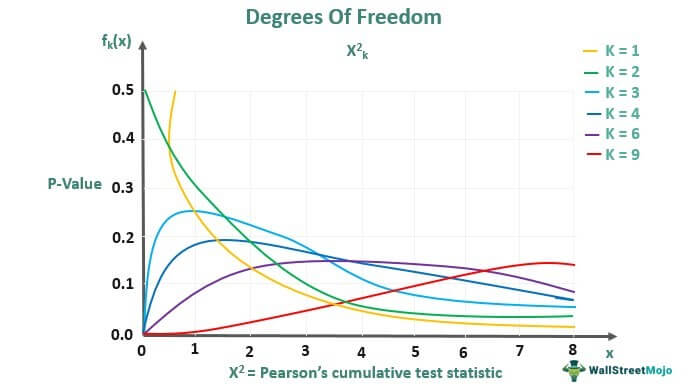
How To Use?Â
To carry out a Chi-square test, one must use the following analysis procedures:
- First, researchers must make a table of expected and observed frequencies.
- Users should specify their null and alternate hypotheses when gathering their data.
- They should then select an alpha value.
- Finally, users must decide how much of a chance they are willing to accept that they will get the incorrect conclusion. For example, consider the scenario when users choose 0.05 as the cutoff for the independence test. In this case, they've calculated a 5% chance that users will mistakenly think the two items are independent variables even if they're not.
- Researchers must then verify the data for mistakes.
- They must verify the test's underlying assumptions.
- Finally, they should run the test using the formula and make a final decision.
- Accept or reject the null hypothesis.