Table Of Contents
What Is Causal Inference?
Causal inference is a process that involves determining the causal relationship that may exist between events or variables by analyzing data or studying how the variables influence each other. In finance, it helps determine what impact regulatory changes can have on the market, whether its stability or liquidity.
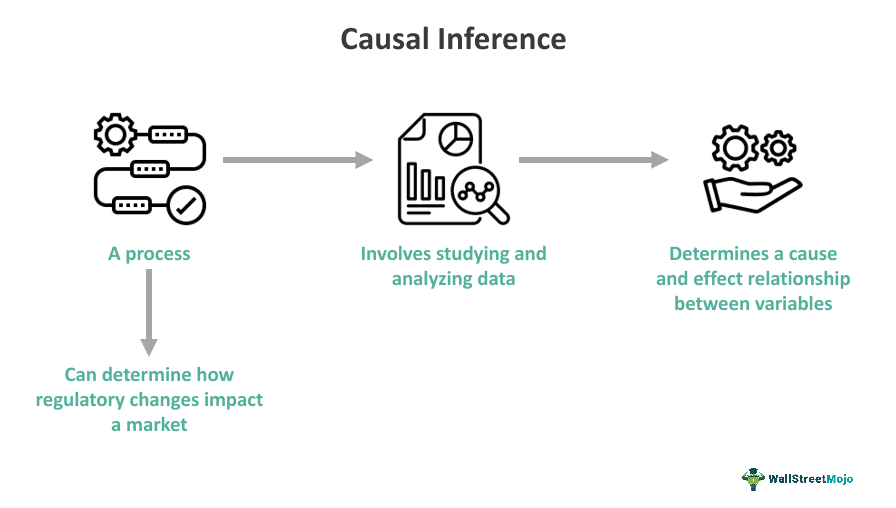
This concept creates a link between decision-making and prediction. This is very useful as even highly accurate prediction models cannot help on their own when reasoning what may happen if one carries out an action or changes a system. Moreover, this process can help in different related situations, for example, when analyzing the effect of intervention or investment.
Key Takeaways
- Causal inference refers to an intellectual discipline that takes into account the study designs, estimation strategies, and assumptions, allowing an observed effect’s underlying source.
- The different kinds of causal inference techniques are experimental, general, and quasi-experimental.
- There are different causal inference assumptions. Three of them are positivity, unmatched confounders, consistency, and unmatched confounders.
- This process offers various benefits in different fields. For example, it helps researchers provide conclusions based on clinical trials and observational data.
- Moreover, it can help businesses comprehend the effect of an advertisement campaign on their topline.
Causal Inference Explained
Causal inference is the derivation of an impact of a certain phenomenon, which is just a part of a larger system, on decision-making, depending on the correlation between data observed. This process helps determine the actual, independent impact of a certain phenomenon, an element of a much larger system. This is a fundamental concept in the fields of epidemiology, economics, statistics, etc.
It establishes a cause-and-effect relation by analyzing data and drawing conclusions regarding how variables influence one another. The data analyzed or studied to draw conclusions regarding causal relationships are observational or experimental.
Causality involves relationships where a change in a factor leads to a corresponding alteration in another factor. Such relationships depend on three factors — the control of the “third variables,” temporal order, and correlation.
Note that it is vital to take into account the chance of spurious relationships, in which the correlation between the variables seems to signal a causal impact but instead effectuates from a shared cause that is hidden.
Assumptions
There are four key causal inference assumptions. Let us look at them in detail.
- Consistency: According to this assumption, the potential income of a person under their observed exposure history is their observed outcome. Note that the experiment design guarantees consistency as the exposure’s application to any person is under the investigator’s control.
- Positivity: Per this assumption, there is a positive or nonzero probability of getting all exposure levels for each combination of exposure values and confounders occurring among persons in the population.
- Unmatched Confounders: According to this assumption, once people condition on the relevant observed confounders, the treatment assignment is separate from the outcomes.
- Stable Unit Treatment Value Assumption: Also known as SUTVA, it assumes that all treated units do not impact other units.
Methods
Some popular causal inference techniques are as follows:
#1 - Experimental
Causal mechanisms’ experimental verification is possible by utilizing experimental methods. The primary motivation behind any experiment is to hold the other experimental variables constant, manipulating the variable of interest purposefully at the same time. Note that if experiments produce significant effects owing to the manipulation of the treatment variables only, there’s scope to think that it is possible to assign causal effects to the treatment variables, assuming the other standards concerning experimental design are fulfilled.
#2 - Quasi-Experimental
Causal mechanisms’ quasi-experimental verification takes place when the conventional experimental techniques are unavailable. This could result from the prohibitive costs incurred for carrying out an experiment. Alternatively, it may result from the inherent feasibility associated with performing an experiment that is concerned with large systems like electoral systems’ economies. Note that quasi-experiments can also take place where the details are withheld for legal reasons.
#3 - General
People use this casual inference technique through the study of systems where one variable or event’s measure is likely to impact another’s measure. In the case of this method, the first step involves formulating a null hypothesis that is falsifiable. One tests it frequently using statistical methods. Frequentist statistical inference refers to the utilization of statistical methods to figure out the likelihood that the data results under this hypothesis by chance.
Experts use Bayesian inference to determine the impact of any independent variable. On the other hand, they typically use statistical inference to compare random variations in the original data or the impact of well-specified causal mechanisms.
Examples
Let us look at a few causal inference examples to understand the concept better.
Example #1
Suppose Jack, a person new to the world of securities trading, sold all ABC shares in his portfolio after the stock price plunged over 6% in a trading session. To identify the cause of the price drop, he had to investigate further. It could be a result of unfavorable news, poor earnings, or regulatory changes announced by the government, etc. That said, Jack concluded that he sold his holdings because of the price drop. He did not take those factors into consideration, which was crucial to reach a conclusion and establish the cause-and-effect relationship.
Example #2
Suppose Samantha, who is an investor, purchased XYZ stock after its price skyrocketed more than 20% over 2 days. She could not find the reason behind the price surge as she did not conduct sufficient research or analysis. The steep rise could be a result of some stellar earnings growth, new partnerships, favorable regulatory changes, etc. That said, Samantha reached the conclusion that she purchased shares of the company because of the increase in price only. She did not consider factors that were essential to indicate a cause-and-effect relationship.
Advantages And Disadvantages
Let us look at the benefits and limitations of this process.
Advantages
- One can use this concept to comprehend the impact of policies and interventions to offer better-improved transparency in automated decisions. Moreover, it can help control the biases in data.
- This concept enables researchers to reach causal conclusions on the basis of observational data or clinical trials.
- It can help predict the result of alterations in variables, which can be helpful in experiments’ designs.
- This concept can help businesses understand the impact of an ad campaign on their sales.
Disadvantages
- Teasing out cause-and-effect relationships in data can be challenging.
- A key threat to this concept is the confounding impact of other variables.
- It cannot offer insights into production relationships that, in many cases, are present in the center of a causal relationship.
Causal Inference vs Statistical Inference vs Prediction
The concepts of statistical inference, prediction, and causal inference can be confusing for individuals who are unfamiliar with them. It is vital for one to understand how they differ to avoid any kind of confusion while understanding the topics. So, let us look at their key differences.
Causal Inference | Statistical Inference | Prediction |
---|---|---|
This process determines whether observed associations reflect a cause and effect relationship. | It is a technique that involves making decisions regarding the parameters of any population on the basis of random sampling. | This is a process that involves utilizing correlations between the variables to hypothesize about future outcomes and events. |
It helps determine the actual, independent impact of a specific phenomenon that is a larger system’s component. | This method enables persons to offer a probable range of values for true values of something within the population. | It helps in forecasting consequences, effects, costs, and outcomes. |