Table Of Contents
What Is Canonical Correlation Analysis?
Canonical correlation analysis (CCA) refers to a technique individuals and organizations can utilize to measure the linear relation existing between a couple of multidimensional variables. It can help people determine the reasons behind general statistical variations between multiple modalities.
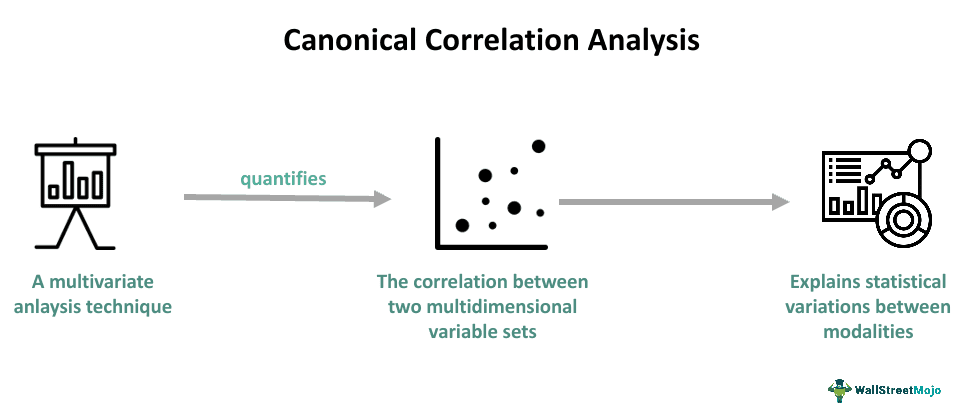
This method finds a couple of bases, one for every variable. Such bases are ideal with regard to correlations. Simultaneously, the technique finds corresponding correlations. The new bases’ dimensionality is less than or the same as the two variables’ smallest dimensionality. Contrary to regression analysis, researchers can find the relation between multiple independent and dependent variables in this case.
Key Takeaways
- Canonical correlation analysis refers to a method exploring the relationships between each variable set and canonical variates.
- Canonical correlation analysis refers to a method exploring the relationships between each variable set and canonical variates.
- Two noteworthy disadvantages of this method are the instability of canonical weight and the difficulty in interpreting the canonical variates that result from this kind of analysis.
- The canonical correlation analysis interpretation is analogous to the interpretation of regression coefficients.
- Canonical correlation in research methodology assumes unrestricted variance. Also, this technique applies to different sectors, like healthcare and finance.
Canonical Correlation Analysis Explained
Canonical correlation analysis refers to a method people use to quantify the correlation between two sets of multidimensional variables; while one of the variables is dependent, the other one is independent. Persons use a statistic known as Wilk’s Lambda to test such a correlation’s significance. Note that the canonical correlation’s work is identical to that of simple correlation. Also, the canonical coefficient interpretation occurs in a way that is analogous to regression coefficients’ interpretation.
As noted above, this analysis involves working with a couple of data sets. That said, instead of factoring in each variable’s correlation with different variables, it involves using a different method. This technique involves a correlation analysis between two data sets’ linear combinations. For instance, let us say that there are a couple of data sets — A and B. Canonical correlations deal with the linear combinations of Y’s and X’s variables utilizing different weights ‘bi.’ Following that, the formation of a correlation between linear combination occurs with ‘Ty’ and ‘Ux.’
Let us understand certain terms associated with this concept to understand it better.
- Redundancy Coefficient (d): It measures the variance percentage of original variables of a particular set predicted from the rest of the sets.
- Canonical Communality Coefficient: Such a coefficient refers to the sum of all squared structure coefficients for a certain variable type.
- Canonical Variate Or Variable: It is a linear combination of the original variables’ set. Such variables fall under the category of latent variables.
- Canonical Weight: Also known as a canonical coefficient, it is first standardized before it is utilized to establish the linear combination that is interpreted in the same manner as a regression coefficient.
- Likelihood Ratio Test: It helps in conducting a significance test of every source of linear relationship existing between a couple of canonical variables.
- Eigenvalues: Eigenvalues’ value in this type of analysis is roughly equal to the value’s square. Basically, the Eigenvalues reflect the variance’s proportion in a particular canonical variate.
Assumptions
The assumptions of canonical correlation in research methodology are as follows:
- A key assumption of this analysis is that variables within a population from where one took the sample must have Gaussian or normal distribution.
- It is not possible to carry out this kind of correlation analysis if one finds multicollinearity among one or multiple sets of variables. Simply put, the variables must not have a correlation of 1 among themselves.
- Similar to multivariate regression, canonical correlation analysis needs a sample that is large in size to create a robust model.
- Unrestricted variance must exist in canonical covariance.
Examples
Let us look at a few canonical correlation examples to understand the concept better.
Example #1
A study investigated the U.S. airline management teams’ perception of the deregulation impact on the financial risk associated with the industry by conducting an analysis of the companies’ risk management behavior. Particularly, canonical correlation analysis helped obtain crucial liability/equity interrelationships. Moreover, the technique allowed for identifying the alterations in the risk management of airlines as indicated by all alterations concerning financial structure.
Per the study results, the U.S. airline industry made adjustments to its financial structure to minimize the risk exposure as it became subject to deregulation. The industry lowered its financial leverage via more equity usage to finance long-term assets while increasing liquidity at the same time.
Example #2
Let's check out this research aimed to empirically spot and explain relations, including the hedging behavior between the capital side and the asset side of large U.S. banks’ balance sheets. Canonical correlation analysis was utilized to fulfill this purpose. The variables utilized in the study were liability or capital and asset categories expressed as the overall bank assets’ proportions.
Such proportions replaced the usual financial rations. Moreover, there was no employment of information exogenous to the financial institutions. The empirical results indicated various relations. For example, the companies utilized hedging, and a few assets served as collateral for factor or short-term bank loans and mortgages.
Applications
Let us look at some real-world applications of CCA:
- Insurance companies utilize this technique to test the relationship between the kinds of insurance policies or products taken, for example, health insurance, life insurance, etc., and individuals’ characteristics, such as age, income, medical background, and gender.
- Many marketers utilize CCA to examine the relationship between consumers’ preferences and demographic factors or various products.
- Credit card companies can conduct this kind of analysis to understand the relationship between the credit cards taken and the type of bank account, for example, savings, current, or fixed deposit.
- Healthcare research centers can use CCA to test the relationship between a disease’s predictors on the basis of a patient’s medical history.
Additionally, people have used this analysis as a statistical tool in meteorology, medical studies, economics etc.
Advantages And Disadvantages
Let us look at the benefits and limitations of canonical correlation.
Advantages
- It helps people interpret the relationship between a couple of variables.
- This tool can help one minimize the size of the computational data available.
Disadvantages
- Interpreting the canonical variates resulting from such an analysis can be challenging. This is because rotation is impossible.
- Canonical weight is associated with a lot of instability.
- This technique reflects only the variance linear composites share. It does not reflect the variances that are extracted from variables.
Canonical Correlation Analysis vs Principal Component Analysis
Some crucial difference between principal component analysis (PCA) and canonical correlation analysis are as follows:
- CCA emphasizes looking for linear combinations accounting for maximum correlation in a couple of datasets. On the other hand, PCA concentrates on searching for the linear combinations that consider the maximum variance in a specific dataset.
- The main objective of PCA is dimensionality reduction, whereas CCA doesn’t primarily aim for dimensionality reduction.