Table Of Contents
What Is A Bivariate Analysis?
Bivariate analysis refers to a process that involves analyzing a couple of variables to know about the relationship between them. It helps in determining whether any correlation exists between the two variables and, if yes, then how strong is that association.
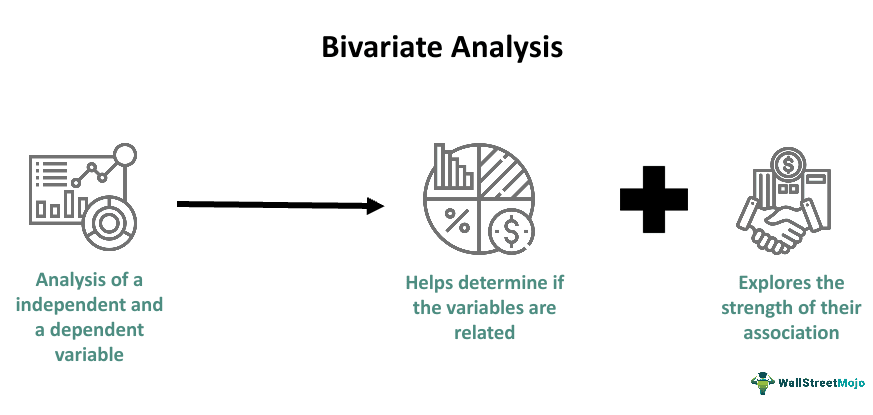
This analysis is useful for making predictions regarding a dependent variable’s value based on the changes to an independent variable’s value. Moreover, it helps in finding patterns and trends in data. There are different types of bivariate analysis, such as numerical and numerical and numerical and categorical. One can use it in different types of research, including medicine, marketing, etc.
Table of contents
- Bivariate analysis refers to a method that involves examining the correlation between an independent and a dependent variable. This type of analysis also determines the strength of the relationship.
- There are three types of this analysis — numerical and categorical, categorical and categorical, and numerical and numerical.
- There are various benefits offered by this analysis. For example, it assists in data pattern and trend identification. Moreover, it helps researchers predict future results.
- A key difference between univariate and bivariate analysis is that the former involves analyzing only a single variable, while the latter involves analyzing two variables simultaneously.
Bivariate Analysis Correlation Explained
Bivariate analysis refers to an analysis of a current correlation involving two attributes or variables. This process gauges the depth of the association between them. This helps in determining if there is any discrepancy between them and the reasons behind it. Moreover, it allows one to refute or verify the association and causality hypothesis.
For this kind of analysis, one must first recognize the bivariate data. In most cases, the data consists of two measurements – X and Y. One can interpret the bivariate data as a pair (X, Y). People often refer to such variables as simple random samples (SRS). One may denote the variables like this – (X1Y1), (X2Y2), (X3Y3), …, (XnYn). Note that usually, people utilize a two-column data table to hold such an analysis’s outcome.
One must not confuse this analysis with a two-sample analysis in which X and Y variables have no direct relation.
Types
Let us look at the different types of this analysis in detail.
#1 - Numerical And Numerical
In this case, both the variables of the bivariable data, including the independent and dependent variables, have numerical values.
#2 - Categorical And Categorical
If both variables of the bivariate data are static, the interpretation of the data takes place and predictions and statements are made regarding it. While carrying out research, this type of analysis helps in determining the impact and cause to conclude that the particular variable is categorical.
#3 - Numerical And Categorical
This type of analysis includes a numerical variable and a categorical variable.
Examples
Let us look at a few bivariate analysis examples to understand the concept better.
Example #1
Suppose Jack needed to study the relationship between two variables — age and systolic blood pressure, in an organization. He had a sample comprising 8 employees aged between 35 and 57 years. The bivariate table below has the results.
Employee (Observations) | Age of Employees | Systolic Blood Pressure |
---|---|---|
1 | 35 | 130 |
2 | 37 | 133 |
3 | 39 | 135 |
4 | 42 | 138 |
5 | 46 | 141 |
6 | 49 | 145 |
7 | 53 | 148 |
8 | 57 | 153 |
He displayed the table graphically using a bivariate analysis technique called scatter plot to reach conclusions. He inferred that there is a positive relationship between the age of the employee and systolic blood pressure. In other words, the older an employee, the older their systolic blood pressure.
Example #2
The COVID-19 vaccines were effective against outcomes and infections. That said, breakthrough infections were increasingly reported owing to emerging variants or vaccine-induced or vaccine-induced immunity. A study aimed to describe the clinical features, time trends, outcomes, and risks of VBT (Vaccine Breakthrough) among patients hospitalized in Egypt.
To spot risk factors concerning VBT, bivariate analysis was utilized for comparing the vaccine type, comorbidity, and demographics between patients without and with VBT. Per the analysis, VBT was more common in younger patients who were 16-35 years old, males, and individuals with diabetes or cardiovascular diseases in comparison to the corresponding groups of people who were not fully vaccinated.
Advantages And Disadvantages
Let us look at the benefits and limitations of bivariate analysis.
#1 - Advantages
- This type of analysis can help spot data patterns and trends by assessing the two variables’ relationship.
- It enables researchers to make predictions by modeling the association of the two variables.
- This analysis can evaluate whether both variables are linked statistically, thus helping researchers establish which variable impacts the other.
- It improves decision-making concerning public policy, business, and healthcare.
#2 - Disadvantages
- Such an analysis only considers the relationship between the two variables. One needs to use multivariate analysis to determine how another variable impacts the others.
- Often, this type of analysis cannot offer any explanation regarding the two variables’ relationship. Instead, it only gives a description. Note that explanatory analysis is mandatory to infer the cause.
Bivariate Analysis vs Univariate Analysis vs Multivariate Analysis
Understanding the concepts of multivariate, univariate, and bivariate analysis can be challenging for individuals new to data analysis. To have a clear idea regarding their meaning and purpose and avoid potential confusion, one must understand their distinct characteristics. In that regard, let us find out how they differ.
Bivariate Analysis | Univariate Analysis | Multivariate Analysis |
---|---|---|
This is a basic form of quantitative analysis | It is the most basic form of statistical analysis. | Such an analysis refers to a statistical technique used to analyze data involving various observations or measurements. |
The primary objective of this analysis is to explain. | Its aim is to describe. | In this case, the main goal is to analyze. |
The comparison of two variables occurs with the use of bivariate statistics. | In this case, the comparison of only one variable occurs using univariate variables. | The comparison of three or more variables takes place through multivariate statistics. |
Frequently Asked Questions (FAQs)
Some popular bivariate analysis techniques are as follows:
- Scatter plots
- Regression analysis
- T-test
- Chi-Square test
- Analysis of variance or ANOVA
- Correlation
This type of analysis can be descriptive, like in the case of scattered plots. That said, typically, the goal of such a method is to compare the relationship of two variables. For instance, researchers may conduct this kind of analysis to figure out if the self-efficacy of students in physics is a key predictor of physics standardized test scores.
Null hypotheses mean that there isn’t any difference between the values in variables 1 and 2, on average.
Bivariate correlations analyze how and if a couple of variables covary linearly, i.e., if the variance of one variable alters in a leaner manner as the other one’s variance changes.
Recommended Articles
This has been a guide to what is a Bivariate Analysis Correlation. We explain its examples, differences with univariate and multivariate analysis, types, and advantages. You can learn more about it from the following articles –