Table Of Contents
What Is Bayesian Information Criterion (BIC)?
The Bayesian Information Criterion (BIC) is a statistical measure that is used in model selection and hypothesis testing to detect the best model among all other available candidates. This method strikes a balance between model fit and complexity and aims to identify the most economical and informative model.
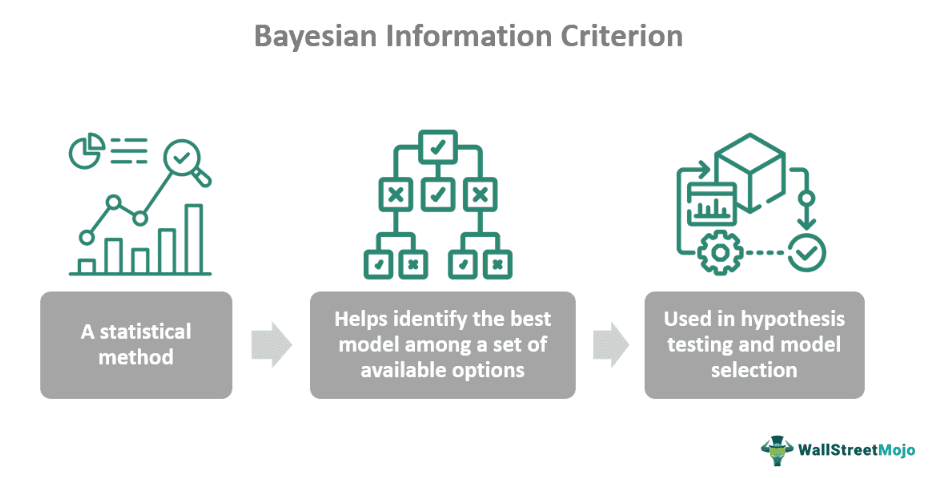
The BIC penalizes models with more parameters. It discourages overfitting by considering both the likelihood of the data and the number of parameters in the model. This process assists in finding the model that best explains the data while avoiding unnecessary complexity.
Key Takeaways
- The Bayesian information criterion is a statistical metric that aids in selecting models and testing hypotheses.
- This approach tries to find the most cost-effective and informative model by striking an equilibrium between model fit and complexity.
- The BIC penalizes models with higher parameters. It prevents overfitting by taking into account both the data's likelihood and the amount of parameters in the model.
- However, the BIC does not take into account the presence of outliers in the data. This approach might not be suitable in circumstances when the presence of outliers can substantially affect the model fit.
Bayesian Information Criterion Explained
The Bayesian information criterion is a crucial tool in statistical modeling, and it provides a systematic approach to selecting the most appropriate model among a set of candidates. The method aids in balancing model complexity and goodness of fit to the data. It helps navigate the complicated trade-off between a model's explanatory power and its complexity.
This method comprises two key elements: the likelihood of the observed data in the model and a penalty term proportional to the number of parameters in the model. This penalty term discourages the inclusion of unnecessary parameters and reduces overfitting. BIC penalizes overly complex models and promotes a more feasible selection. A lower BIC value signifies a more favorable compromise between model fit and simplicity. The method leads analysts to a model that avoids the risks of excessive complexity and accurately reflects the fundamental structure of the data.
Characteristics
The characteristics of the Schwarz Bayesian Information Criterion are:
- It is designed to maintain a balance between the goodness of fit to the observed data and the model complexity. The method achieves this by penalizing models with more number of parameters. This discourages overfitting and favors simpler models that generalize well to new data.
- BIC incorporates the sample size in its penalty term. A larger sample size leads to a more substantial penalty for additional parameters. This reflects the idea that as the amount of data increases, the penalty for model complexity should be adjusted accordingly.
- The BIC is a versatile method that is applicable to a wide range of statistical models, including linear regression, time series models, and more. Its flexibility makes it a widely used tool in diverse disciplines like economics, biology, and machine learning.
Formula
The Bayesian Information Criterion formula is as follows:
Bayesian Information Criterion formula = k log(n)- 2log(L(θ))
Where,
n = the sample size
k = the number of parameters that the model estimates
L(θ) = the maximized value of the likelihood of the model tested
Examples
Let us study the following examples to understand the BIC:
Example #1
Suppose James is an analyst who wants to compare two models with the BIC. He starts calculating the BIC for each model. James has a set of data with 100 observation points. Model 1 estimates three parameters, while Model 2 estimates four parameters. Suppose the log of maximum likelihood for model 1 is a, and for model 2, it is 2a. Using the formula k log(n)- 2log(L(θ)):
Calculating BIC on this data gives the following:
- Model 1: 3log(100) – 2a = 6 – 2a
- Model 2: 4log(100) – 4a = 8 – 4a
This is a Bayesian Information Criterion example.
Example #2
Placental dysfunction, the underlying cause of frequent pregnancy disorders including preeclampsia, fetal development restriction, and spontaneous premature delivery, is still not fully comprehended. These prevalent obstetrical disorders display comparable placental histopathologic patterns despite their clinical differences. Individuals within each syndrome exhibit unique molecular modifications, which complicate issues and make it more challenging to prevent and cure these syndromes.
A study comparing the relationships between SNF clusters, illness states, and histopathological characteristics was carried out using Bayesian model selection. The findings showed that integrated omics-based SNF increases our understanding of the pathogenic processes and distinctively redefines placental dysfunction patterns causing common obstetrical disorders. This is another Bayesian Information Criterion example.
Limitations
Some limitations of the Schwarz Bayesian Information Criterion include the following:
- It assumes that the errors in the model are normally distributed. In cases where this assumption is violated, the method may not provide accurate results.
- This measure is not suitable for comparing non-nested models. Comparing non-nested models requires alternative criteria, and BIC may not provide meaningful comparisons in such situations.
- It tends to provide a single model as the best choice and neglects uncertainty associated with model selection. However, in reality, there may be several models with similar support, and BIC does not account for this uncertainty.
- The BIC does not account for the presence of outliers in the data. In situations where outliers can significantly influence model fit, this method may not be appropriate.
Bayesian Information Criterion vs Akaike Information Criterion
The differences between Bayesian and Akaike Information Criterion are as follows:
Bayesian Information Criterion
- BIC penalizes models heavily for increased complexity by incorporating a logarithmic term that is proportional to the number of parameters in the model.
- The penalty term has a more substantial bias towards simpler models. This attribute makes it particularly useful in situations where overfitting is a significant concern.
- BIC functions under the assumption that the actual model is among the candidate models in the study.
- BIC is sensitive to sample size, with a more substantial penalty for complexity in smaller datasets.
Akaike Information Criterion (AIC)
- AIC penalizes models for complexity less severely. It includes a term that is linearly proportional to the number of parameters.
- It strikes a balance between model fit and complexity and is less biased towards simplicity.
- AIC is more robust in situations where the actual model may not be present.
- This measure is generally less sensitive to sample size and is a preferred choice in situations with limited sample sizes.