Table Of Contents
What Is Augmented Dickey-Fuller Test?
The Augmented Dickey-Fuller (ADF) test is a statistical test used in econometrics and finance to determine whether a unit root is present in a time series dataset. A unit root implies that a series is non-stationary, i.e., its statistical properties, such as mean and variance, are not constant over time. In financial terms, non-stationary data can exhibit trends or cycles that make it challenging to model and forecast accurately.
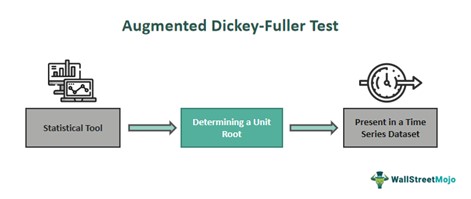
The ADF test in finance serves the purpose of assessing the stationarity of a financial time series. This includes stock prices, interest rates, or exchange rates. Stationarity is essential for reliable financial modeling and forecasting. It ensures that the statistical properties of the data remain constant over time. The ADF test aids analysts in determining whether a unit root is present. This understanding helps in assessing whether a financial series is likely to revert to its mean or exhibit long-term trends.
Key Takeaways
- The Augmented Dickey-Fuller test is used to determine whether a time series dataset is stationary or non-stationary.
- It helps identify the presence of a unit root in the data, indicating non-stationarity.
- By comparing the test statistic to critical values, the ADF test allows for statistical inference about the stationarity of the series.
- The ADF test finds widespread application in finance for analyzing financial time series data, building predictive models, testing asset pricing theories, and risk management.
Augmented Dickey-Fuller Test Explained
The Augmented Dickey-Fuller (ADF) test, originating from the works of Dickey and Fuller in 1979, is a fundamental tool in finance for assessing the stationarity of time series data. Its roots lie in the field of econometrics, specifically in the study of unit roots and stochastic processes. In finance, the ADF test serves as a cornerstone in analyzing the behavior of financial variables over time.
From a financial perspective, the ADF test is akin to a diagnostic tool for detecting the presence of trends or cycles in financial time series. It aims to uncover whether a financial variable tends to revert to a long-term mean or if it perpetually diverges from it. This distinction is crucial for understanding the predictability and risk associated with financial assets.
By determining the stationarity of financial data, the ADF test aids in identifying patterns that can inform investment strategies, risk management techniques, and the development of predictive models. Its application extends across various financial domains, including asset pricing, portfolio management, and derivative pricing, making it an indispensable tool for financial analysts and researchers alike.
Assumptions
The Augmented Dickey-Fuller (ADF) test relies on several key assumptions to ensure the validity of its results:
- Stationarity: The ADF test assumes that the underlying financial time series is stationary, meaning its statistical properties, such as mean and variance, remain constant over time. Stationarity is crucial for reliable inference because it ensures that the relationship between variables remains consistent throughout the data.
- Absence of serial correlation: The test assumes that there is no autocorrelation present in the residuals of the model. Serial correlation, or the dependence of one observation on previous observations, can distort the results of the test and lead to incorrect conclusions about the stationarity of the series.
- No structural breaks: The ADF test assumes that there are no structural breaks or sudden changes in the underlying data-generating process over the period under consideration. Structural breaks can invalidate the test results by introducing non-stationarity into the series.
- Adequate pattern size: The test assumes that the sample size is sufficiently large to provide reliable estimates of the parameters in the model. A small sample size can lead to unreliable test results and increased uncertainty in the conclusions drawn from the test.
Examples
Let us understand it better with the help of examples:
Example #1
Suppose we want to determine the stationarity of a stock price series. We collect daily closing prices of a particular stock over the past five years. We then conduct an Augmented Dickey-Fuller (ADF) test on this dataset. The null hypothesis of the test is that the stock price series has a unit root, indicating non-stationarity. If the test statistic is less than the critical value at a chosen significance level (e.g., 5%), we reject the null hypothesis and conclude that the stock price series is stationary. Conversely, if the test statistic is more significant, we fail to reject the null hypothesis, suggesting non-stationarity.
Example #2
Recent research published in "ScienceDirect" in 2022 sheds light on economic trends. The study, led by renowned economists, employs the Augmented Dickey-Fuller (ADF) test to analyze global economic indicators. Surprisingly, the findings suggest a significant shift in long-term trends, challenging conventional economic wisdom. Notably, the ADF test unveils previously unnoticed patterns, prompting economists to reevaluate forecasting models and policy implications. The study underscores the importance of robust statistical tools like the ADF test in understanding complex economic dynamics, offering fresh insights for policymakers and investors alike.
Application In Finance
The Augmented Dickey-Fuller (ADF) test holds significant application in finance, primarily in analyzing and forecasting financial time series data. Here's how it's applied:
- Testing for Stationarity: A critical application of the ADF test in finance is to assess whether a financial time series is stationary or not. Stationarity is crucial for reliable financial modeling and forecasting. By determining whether a unit root is present in the series, the ADF test helps analysts understand if the series exhibits long-term trends or if it reverts to a mean over time.
- Building Predictive Models: In finance, accurate predictions are essential for making informed investment decisions. The ADF test helps analysts identify the appropriate transformations or models to apply to non-stationary data to make it suitable for forecasting. This ensures that predictive models provide reliable estimates and insights into future market movements.
- Risk Management: Non-stationary financial series can pose challenges for risk management strategies. The ADF test assists in identifying trends or cycles in financial data, enabling risk managers to adjust their strategies accordingly to account for potential market fluctuations.
- Asset Pricing: The ADF test is also used in asset pricing models to determine the relationship between financial variables and asset prices. By examining the stationarity of relevant variables, analysts can better understand the pricing dynamics of financial assets.
Augmented Dickey-Fuller Test vs Dickey-Fuller Test
Augmented Dickey-Fuller Test
- An augmented version of the Dickey-Fuller test.
- Handles higher-order autocorrelations more effectively.
- Allows for the inclusion of additional lagged terms in the model.
- More commonly used in practice due to its improved performance.
- Provides more robust results for testing stationarity in time series data.
- Suitable for analyzing larger datasets with potentially complex autocorrelation structures.
- Widely adopted in econometrics and finance for testing stationarity.
Dickey-Fuller Test
- The original version of the Dickey-Fuller test.
- Limited in its ability to handle higher-order autocorrelations.
- Relies on a simpler model with fewer lagged terms.
- Less commonly used compared to the augmented version.
- It may produce less reliable results, especially with more complex data.
- Best suited for simpler datasets with fewer autocorrelation issues.
- Historically significant but less prevalent in contemporary analysis.